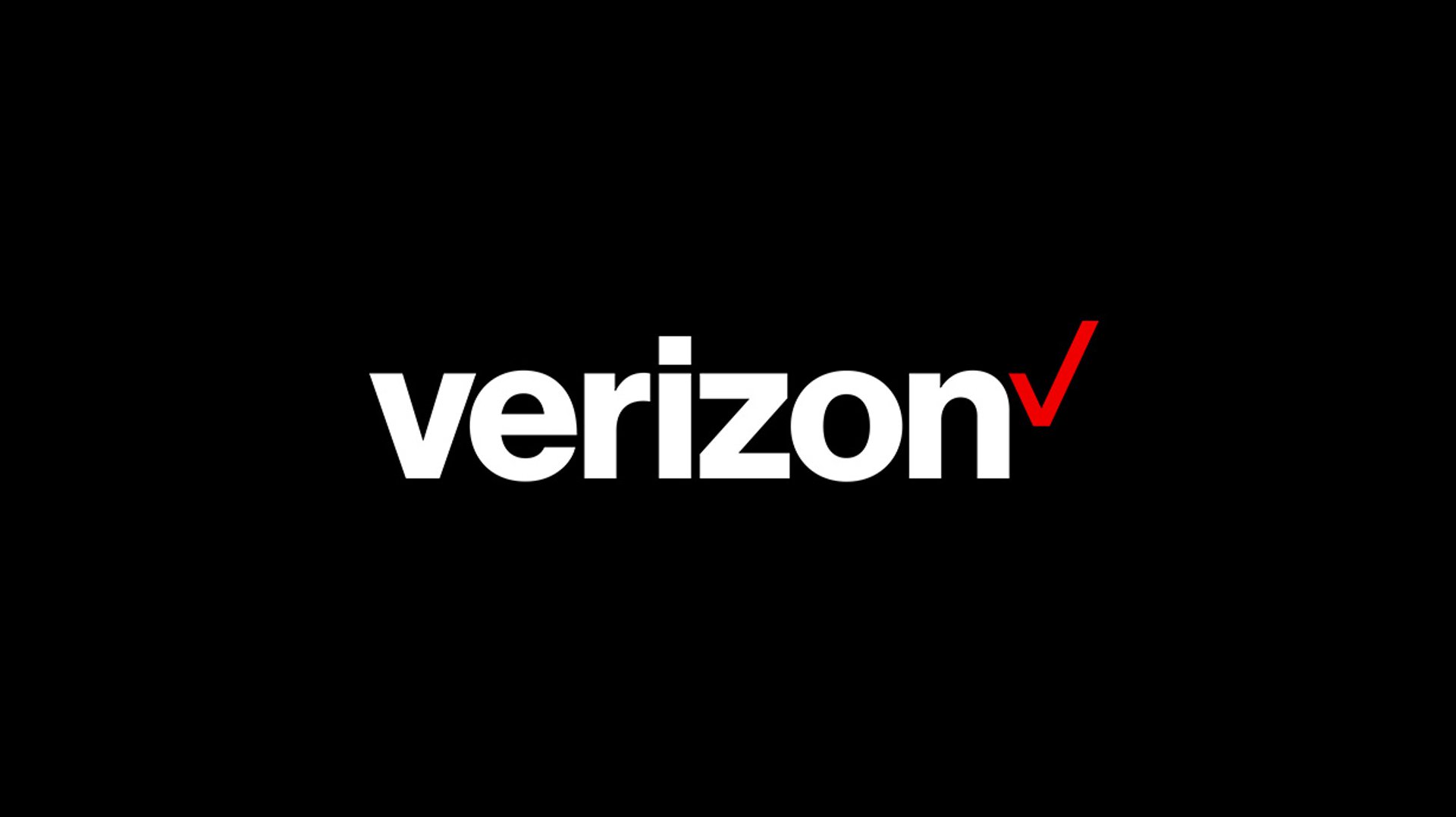
Case Study: The Verizon Contact Center Improved Customer Experience While Strengthening Security Measures.
Executive Summary:
This study demonstrates how Verizon's contact center uses AI systems to lower fraud instances while increasing revenue streams, resulting in improved customer security and experience.
Product Manager Contributions:
As the Product Manager, I organized discovery sessions with business stakeholders at the contact center to understand their challenges and requirements. Throughout the project, I led cross-functional teams to maintain their cooperation and alignment towards shared goals. My design of IVR user flows improved customer engagement and interactions.
To define project requirements in detail, I generated epics alongside user stories and acceptance criteria. Through team collaboration with engineers and developers, I directed backlog management to achieve technical viability and feature delivery deadlines, which led to a successful AI-driven fraud detection system deployment.
Customer Flow for Voice Online Fraud Detection:
• Customer Initiation: Customers make contact through multiple channels, including phone calls and online methods.
• AI Analysis:
o Action: The AI system analyzes both transaction details and associated options, including flags and patterns.
o Outcome: Evaluate customer sentiment while identifying potential fraud indicators.
• Insights & Orchestration:
o Action: The system extracts actionable insights about customer interactions and possible fraudulent activities.
o Outcome: Tailor responses for personalized service.
• Agent Response:
o Action: Agents obtain direction through AI-generated insights and information about customer interactions.
o Outcome: Enhanced decision-making for fraud prevention.
• Feedback Loop:
o Action: Collect customer feedback post-interaction.
o Outcome: Improve AI model accuracy with real-time updates.
Data Flow for Voice Online Fraud Detection:
• Data Collection:
o Source: Compile information from phone calls, together with online transactions data and interactions with customers.
• Data Processing:
o Action: Process analyzed data to identify fraud patterns.
• Surveillance & Security:
o Action: Keep track of flagged interactions to detect suspicious behavior and confirm data accuracy.
• Alert & Insight Generation:
o Action: Create alerts to identify potential fraud cases along with insights that agents can use.
• Data Utilization:
o Action: Agent actions should be guided by information, which will enhance fraud prevention strategies.
Customer and Business Outcome:
• Reduced Fraud Losses: Data-driven AI-based insights enabled the organization to reduce annual fraud losses by $2 million, alongside a 15% increase in revenue.
• Improved Retention: The improvement of IVR and anti-fraud systems led to a rise in customer retention by 20%, along with a $1.5M enhancement in Annual Recurring Revenue (ARR).
Conclusion:
Verizon's contact center saw major improvements in fraud detection capabilities and customer satisfaction after AI implementation, which resulted in better security and operational effectiveness. The initiative achieved a $2M annual reduction in fraud losses while boosting revenue by 15% through AI-based data analysis, and it enhanced customer retention by 20% as well as increased ARR by $1.5M through upgrades to IVR and anti-fraud systems.
Reflection:
The example demonstrates AI's role in revolutionizing customer service and security operations through a proactive strategy for managing fraud in the telecommunications sector while exemplifying that most business problems can be solved with a teamwork approach.